A Tutorial on Parent-Child Relationship in Marketing Cloud Intelligence
Understanding Overarching Entities in Datorama
Overarching entities in Datorama play a crucial role in classifying data shared across multiple streams within a data model, including search, social media, web, and programmatic sources, thereby providing seamless unification.
Essentially, this ensures that sales data, plan data, and media insights all integrate seamlessly into a single, cohesive structure in Datorama.
Datorama can help unify web analytics with conversion data or Facebook ad insights.
However, not all fields align seamlessly across data streams.
By offering overarching entities that ensure your workspace stays organised while all points align without duplication or confusion.
How Datorama Handles Custom Classifications
The relationship between custom classifications and overarching entities in Datorama.
Custom classifications function as attributes of your central entity.
For instance, if the entity is Media Buy, then custom classification becomes its defining feature, ensuring clarity and preventing data mismatches in your streams.
Datorama’s custom classifications can serve a multitude of functions.
From linking media buys with specific brands or devices to matching device categories with ad data, these classifications ensure consistency across entities such as browser type, geolocation, or product category.
Each attribute plays its part by providing clarity regarding qualities associated with an entity, such as browser type or geolocation information.
Workspace-Level Entities in Datorama
A key highlight of Datorama is its management of workspace-level entities.
These entities exist independently of the data stream that introduced them.
Even if you delete a data stream, the overarching entities remain intact.
This design ensures that classifications remain consistent and accessible, preventing accidental data loss.
For instance, attributes such as device category, browser, geo-targeting, or product details are tied to your workspace, not the individual streams.
This gives you flexibility in managing your data without worrying about disrupting the overarching structure Datorama provides.
Mapping Relationships in Datorama
Let’s examine mapping relationships within Datorama.
A fundamental rule states that every custom classification must have one-to-one relationships with its central entity.
For instance, media buy keys are linked exclusively with one brand without overlaps or multiple values being present.
Datorama can facilitate complex data mappings efficiently while remaining clear through its structure, thanks to entities with many-to-many relationships, such as device categories or geolocations, which feature many attributes per entity.
With such flexibility at their disposal, Datorama can effectively handle intricate mapping processes while maintaining a structured approach.
Combining Data Streams in Datorama
Datorama makes connecting diverse data streams easy, think advertising datasets with media buy keys or social data with post-level details.
All it takes to unite these streams for actionable insights is finding common ground between them.
Datorama helps unify data streams by mapping attributes such as device categories or brands across them.
For instance, mapping an attribute associated with a media buy key to an attribute within an ad dataset.
Similarly, web analytics data can match up with media insights by recognising shared attributes, making Datorama invaluable when it comes to unification efforts.
Understanding Datorama for Brand Mapping
Working with Web Analytics data often presents me with the challenge of mapping brands to media using custom attributes.
At first, this seems straightforward: connect the dots between brand and media using custom attributes, and everything should fall into place.
However, when I check my Web Analytics data stream, I find no trace of media by custom, which creates roadblocks.
Without media by custom attributes in Web Analytics, my plan quickly unravelled.
Since they don’t exist there, my plan collapsed into chaos.
That’s where Datorama proved invaluable. Datorama allows me to implement custom classifications that cover all data stream types.
Initially, I extracted the brand from Web Analytics and mapped it to custom classification one.
Simultaneously, I performed the same operation for ads by mapping both to this same custom classification.
Custom classification serves as my bridge across different datasets by consistently representing my brand column across them all.
When combined, the columns from media by campaign and Web Analytics merge seamlessly.
Datorama makes classification simple.
Once I provide Datorama with my list of brand categories, they ensure that each brand is classified accordingly, whether using ABC or ABB classification, to provide clarity and consistency across my data sets.
Now it is crucial to realise Datorama allows mapping at multiple levels.
I have previously established that brands are typically attributes of media by key, with direct ties connecting their custom classification to this relationship.
Furthermore, each attribute will require its classification sheet so that all data points are appropriately categorised within Datorama’s structure.
Practically, I utilise custom classification one level one as an additional layer of classification.
Integrating all three data sets within Datorama enables me not only to classify but also to maintain hierarchical relationships within my data.
Datorama’s classification system makes the transition seamless, and I can categorise all data streams effectively within its umbrella framework.
With Datorama’s seamless management of classifications, my analytics workflow becomes streamlined and indispensable.
Understanding Parent-Child Relationships in Datorama
Datorama’s parent-child feature can be an indispensable asset.
Imagine two data streams that need to merge but lack a common thread.
Datorama’s parent-child feature helps join these streams based on shared dimensions, seamlessly cutting measurements and dimensions across all dimensions.
How to Merge Data Streams in Datorama
Imagine two data streams sharing similarities, such as media by key.
Datorama enables us to combine them into a single central pool of data by merging both streams into an aggregate parent-child structure, which simplifies the merging process.
By uploading datasets containing details such as media by colour, tactic, and impressions to Datorama’s platform, you can effortlessly map these attributes, turning complex raw data into actionable insights.
Mapping Attributes in Datorama
Datorama offers exceptional mapping capabilities.
When I want to map unique attributes, such as media by colour, using custom attribute fields, they are indispensable for accurately assigning these unique identifiers to my shared data pool.
Aligning all dimensions ensures accuracy. Datorama offers model field properties to further refine my data.
I find changing display names of attributes, such as media by colour, easier for reporting and visualisation without impacting aggregate accuracy.
Visualising Data with Datorama
Datorama makes visualising data simple. I found it effortless when merging streams without proper mapping.
However, by setting default values during data harmonisation, Datorama ensures all dimensions and metrics align seamlessly and create coherent visuals.
Datorama provides tools that enable me to combine datasets of clicks and impressions, along with media by colour, for analysis purposes.
I regularly utilise Datorama’s platform to refine and verify our data for more in-depth, insightful analyses.
Advanced Features in Datorama
Datorama goes beyond simple merging; it optimises.
By exploring parent-child relationships, I’ve managed to structure data in ways that eliminate redundancies while improving clarity.
Attributes such as custom fields and model properties have also enhanced this experience.
Datorama provides me with flexibility when managing text, dates and images within datasets.
With its model field properties, I can fine-tune data accuracy across reports.
Understanding Hierarchy in Datorama
Datorama excels at organising data hierarchically, data modelling professionals know this well.
At Datorama, data is organised with complete hierarchy classification systems in mind to ensure media buys, campaigns, and sites can all be appropriately classified.
Datorama excels at managing complex data streams, including entire hierarchies that encompass media buys, campaigns, and site elements.
This allows us to structure parent-child relationships for greater business efficiency.
Datorama recognises that data streams with entire hierarchies tend to outshine those with lesser or no hierarchy.
Accordingly, we refer to those data streams with complete hierarchies as parents, while any lacking hierarchies are referred to as children.
Parent-Child Relationships in Datorama
Datorama follows an inheritance model, where children inherit some or all of their parents’ attributes.
When a data stream includes both the media buy key and name, as well as the campaign key, name, and site key, along with site name information.
It takes precedence over those that only contain this data, such as media buy key-only or media name-only data streams.
Datorama uses default values when child data streams lack campaign or site values to maintain clean mapping within Datorama.
When mapping occurs in Datorama without error, this ensures an efficient process.
Datorama data structures cannot tolerate data streams with limited hierarchies taking control of an entirely hierarchical one, otherwise, errors and incorrect values will inevitably appear, dissolving their data structure altogether.
Setting Up Parent-Child Relationships in Datorama
Establishing parent-child relationships within Datorama requires careful assessment.
Consider two data streams, one with complex hierarchical structures and another that only contains media buy key and name pairs.
Utilising Datorama’s parent-child feature, we identify an overarching hierarchical data stream as its parent to facilitate seamless attribute integration across datasets.
Datorama takes excellent care in mapping parent-child relationships correctly, and values will flow smoothly as expected if we match media buys with each other correctly.
Applying Join Operations in Datorama
Datorama works similarly to Excel when setting up parent-child relationships by mapping IDs across datasets to ensure a proper hierarchy flow.
Joins are central to Datorama’s set-up. Parent-child relationships serve as full outer joins that bring all relevant data together into an organised structure.
Parent-child joins are essential in maintaining the hierarchy within Datorama and ensuring that media buy keys are aligned correctly, thereby preventing data loss or incorrect default values from taking effect.
Visualising Parent-Child Data in Datorama
Once parent-child relationships are set up in Datorama, data visualisation tools provide a quick way to explore them via media buy keys, attributes, impressions, and clicks.
Incorporating Datorama’s visualisation tools further ensures all attributes, tactics and colours are correctly placed within their hierarchy.
Given that Datorama doesn’t enforce hierarchy automatically, we need to apply strategic structuring techniques to assign appropriate parent-child relationships and analyse accurate data sets.
Exploring Different Parent-Child Scenarios in Datorama
Datorama enables us to easily establish “parent-child relationships within entities,” aligning data streams for optimal performance.
Another approach in Datorama is “parent-child mapping across datasets”.
By creating hierarchies within datasets, we ensure effective structuring.
With Datorama’s Parent-Child feature, media buy keys, campaign attributes, and site relationships can be managed effortlessly for accurate analysis and reporting.
Understanding Datorama’s Parent-Child Relationship
Datorama simplifies data management with parent-child relationships, allowing for easy organisation of data streams by editing child streams directly, rather than editing parent streams.
When editing, always focus on editing the child rather than the parent streams when working with streams of information.
Let’s consider an example in which PC1 has been assigned as the parent and PC2 as its child; then, adjustments made through Datorama need to be refreshed before their changes take effect.
Hierarchies in Datorama
Hierarchy plays an essential role in Datorama. Our data sets consist of media key, campaign name and impression data sets, while another one houses media key and click data sets.
Parent datasets must have the most inclusive hierarchy to ensure accurate classification.
A misplacement could cause misalignments, default values and data inconsistencies to arise.
Editing Parent-Child Assignments in Datorama
Datorama provides a tool for easily changing parent-child relationships by allowing you to select an alternative parent for any given entity.
Incorrect hierarchies can result in missing attributes, causing default values to be applied in their place.
As soon as a hierarchy error arises, corrective action such as revising parent-child relations and refreshing ensures proper classification and data integrity.
Applying Hierarchy Across Entities in Datorama
Parent-child relationships across entities in Datorama operate similarly and should be carefully managed, taking into account the hierarchy.
Data sets containing media key and campaign key dimensions must also be structured accurately for optimal use.
Before creating relationships in Datorama, always determine your components and hierarchy to avoid fragmented or inconsistent data sets. If necessary, consult an expert outside of your organisation.
The Importance of Hierarchy in Datorama
Hierarchy remains at the core of data processing at Datorama, from categorisation through to distribution and storage.
Parent-child relationships help organise data effectively for an ordered approach.
Datorama emphasises hierarchy when conducting operations such as dimension overrides and entity attribute updates, further increasing data clarity and organisation.
Essential Factors in Parent-Child Structure
Focus on setting up parent-child relationships on Datorama by considering three key components: data components, standard keys, and hierarchy.
Understanding these components is integral in building strong relationships and maintaining well-defined data flows.
Validating Parent-Child Setup in Datorama
Verification is key in creating successful parent-child relationships in Datorama.
Always cross-check data associations and relationships using pivot tables for optimal alignment.
Datorama provides continuous validation to minimise errors and maintain structured data, making it a helpful solution for hierarchical data management.
Understanding Data Streams in Datorama
Are You Working with Datorama and Wondering Why Your Data Is Updating Automatically?
Let’s Make This Clear
Datorama can automate the quick upload of Google Ads data.
Perhaps your account manager provides it to you directly, or you need to upload it via direct downloads from Datorama. Datorama provides efficient uploads.
Uploading data directly to a single stream, rather than creating multiple streams, ensures consistency when processing files.
Instead of recreating new streams when updating files, updates are applied to existing streams, which keeps the hierarchy intact and saves time when updating datasets.
Maintaining Hierarchies in Datorama
Datorama operates using hierarchies, meaning every time you add or alter columns or data structures, Datorama must properly map them to their hierarchy.
Whenever changes are made, such as adding or removing columns from Datorama’s mapping system, revisions must be made so that it recognises their new hierarchy structure.
Google Ads data has a well-established structure, with campaign, ad group, keywords, impressions, and clicks being just a few examples.
When transitioning from June data to July data, update it within its current hierarchy without altering the dataset’s appearance.
Handling Attribute Updates in Datorama
Datorama operates using hierarchies, so anytime you introduce new columns or modify data structures, they must be mapped adequately into Datorama’s schema.
If any columns are removed or added back in later, Datorama must adjust to reflect this new hierarchy by revoking any previously set mappings that don’t match its new look.
Google Ads data has an established structure: campaigns, ad groups, keywords, impressions, and clicks are tracked over time in each month without alteration to this structure.
When transitioning between June and July data, update it under its original hierarchy without making significant modifications.
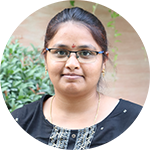
Sai Susmitha
Author